Other digital twin solutions include advanced technologies, systems, and concepts that do not fit into traditional digital twin applications but are key to their further development. They can include simulation of social processes, management of critical infrastructure, digital predictive models for entire cities (smart cities), and integration of digital twins with future technologies, such as quantum computing or the use of biosensors to monitor biological processes.
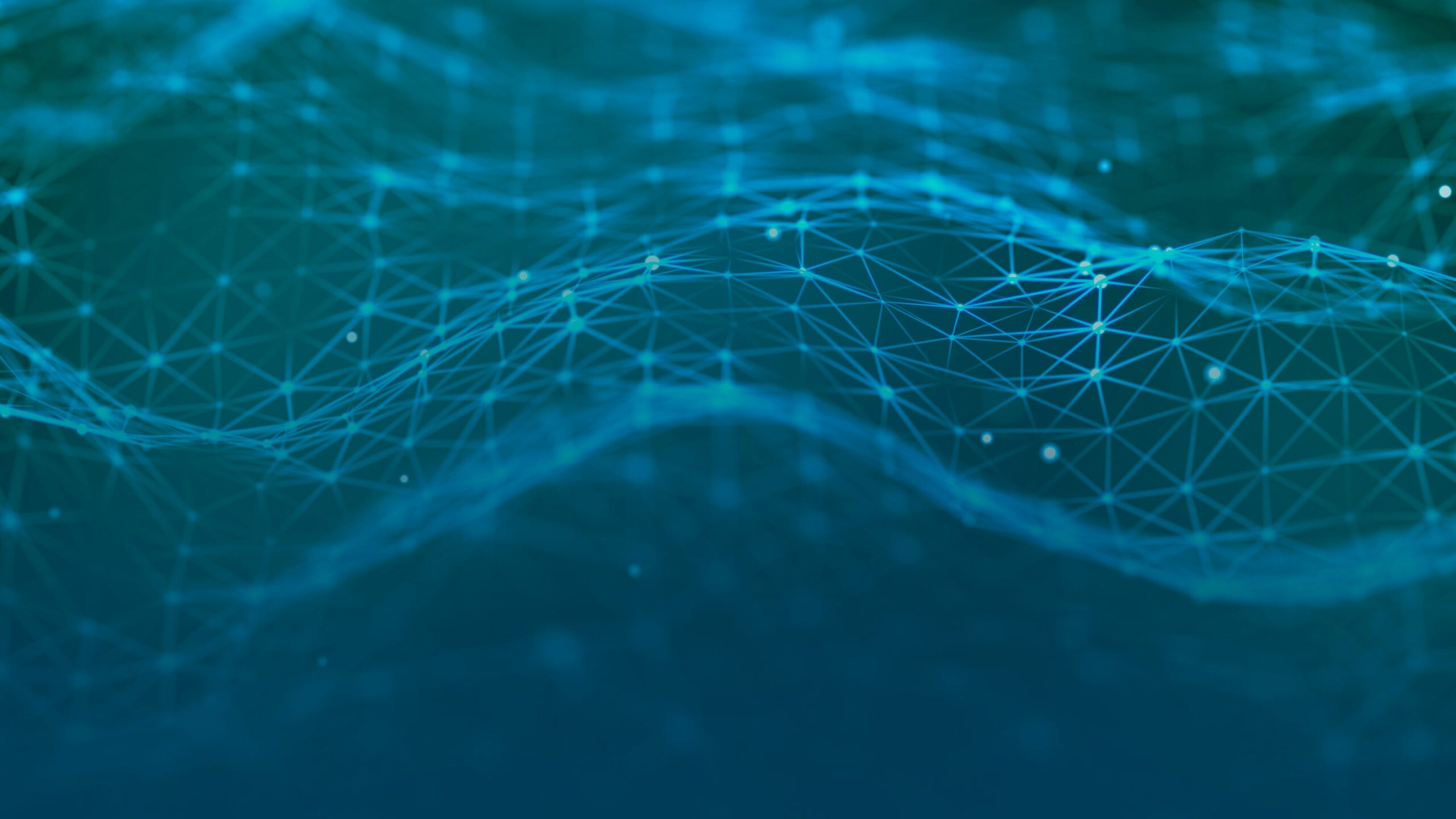
Other Digital Twin Solutions
Type of technology
Description of the technology
Basic elements
- Models of complex processes: Representation of interactions in complex systems, such as cities or logistics networks.
- Crisis management systems: Digital models for emergency planning and response.
- Energy management: Predictive models and simulations of energy consumption under various conditions.
- Biological simulation: Digital representation of biological processes, such as the behaviour of organisms.
- Infrastructure monitoring systems: Tools for monitoring and predicting failures in critical infrastructure.
Industry usage
- Smart cities governance: Digital predictive models for entire cities.
- Energy industry: Simulations of energy consumption and grid management.
- Transport and logistics: Optimisation of transport flows and monitoring of infrastructure condition.
- Critical infrastructure: Monitoring and predicting failures in power and water supply networks.
- Medicine and biotechnology: Digital representation of biological processes for research.
Importance for the economy
Other digital twin solutions support advanced management of complex systems, such as cities, power grids, and logistics processes. With these technologies, it is possible to accurately model complex relationships, predict problems, and optimise the performance of large systems. This is crucial for effective resource management, environmental protection, and the security of critical infrastructure.
Related technologies
Mechanism of action
- Other digital twin solutions rely on the integration of multiple data sources and the use of advanced simulation models. Depending on the application, these models can take into account environmental variables, demographics, biological parameters, or energy flows. The creation process uses technologies such as AI, Big Data, and IoT to create dynamic and complex simulations. For example, predictive models for smart cities can predict energy consumption, traffic, and potential environmental risks.
Advantages
- Managing complex systems: Optimising complex processes in cities and logistics networks.
- Predicting risk: Identification of threats and quick response to changes in systems.
- Resource optimisation: Efficient use of energy, water, and other resources.
- Cost reduction: Minimisation of operating costs through precise management.
- Better planning: Development planning based on accurate predictive models.
Disadvantages
- High implementation costs: Significant investment in infrastructure and specialised software.
- Cybersecurity risks: Possibility of a security breach of sensitive data.
- Technological complexity: Difficulties in integrating different technologies and systems.
- Lack of standardisation: Difficulties with interoperability between systems from different providers.
- Lack of competence: Shortage of specialists capable of designing and maintaining complex systems.
Implementation of the technology
Required resources
- Simulation software: Tools for creating complex models and scenarios.
- Spatial and demographic data: Data sets on location, population, and environmental conditions.
- Data integration systems: Tools for combining data from different sources.
- Computing servers: High-performance units for processing large volumes of data.
- Teams of analysts: Specialists responsible for analysing and visualising complex data.
Required competences
- Systems engineering: Design and implementation of complex simulation systems.
- Modelling and simulation: Creation of models of complex processes and systems.
- Data analysis: Processing and analysing large data sets from multiple sources.
- Cybersecurity: Protecting systems from cyber threats.
- Project management: Coordination of projects involving multiple technologies.
Environmental aspects
- Energy consumption: High energy demand of computing systems.
- Emissions of pollutants: Indirect emissions from the operation of advanced IT systems.
- Raw material consumption: High demand for electronic and computing components.
- Recycling: Problems with recovery of raw materials from advanced IT equipment.
- Waste generated: Electronic waste from equipment replacements and upgrades.
Legal conditions
- Data protection: Regulations for the processing and storage of sensitive data.
- Intellectual property: Patents for simulation technologies and predictive models.
- Critical infrastructure security: Standards for protecting infrastructure from threats.
- Export regulations: Export regulations for advanced simulation systems.
- Environmental protection: Regulations on the environmental impact of advanced IT systems.