The infrastructure for creating a digital twin includes the technical resources and systems needed to build virtual replicas of real objects, processes, or systems. It consists of high-performance computers, IoT sensors, communication systems, analytical platforms, and specialised simulation and modelling software. This type of infrastructure enables real-time data collection, analysis, and mapping of dynamically changing operating conditions of the object.
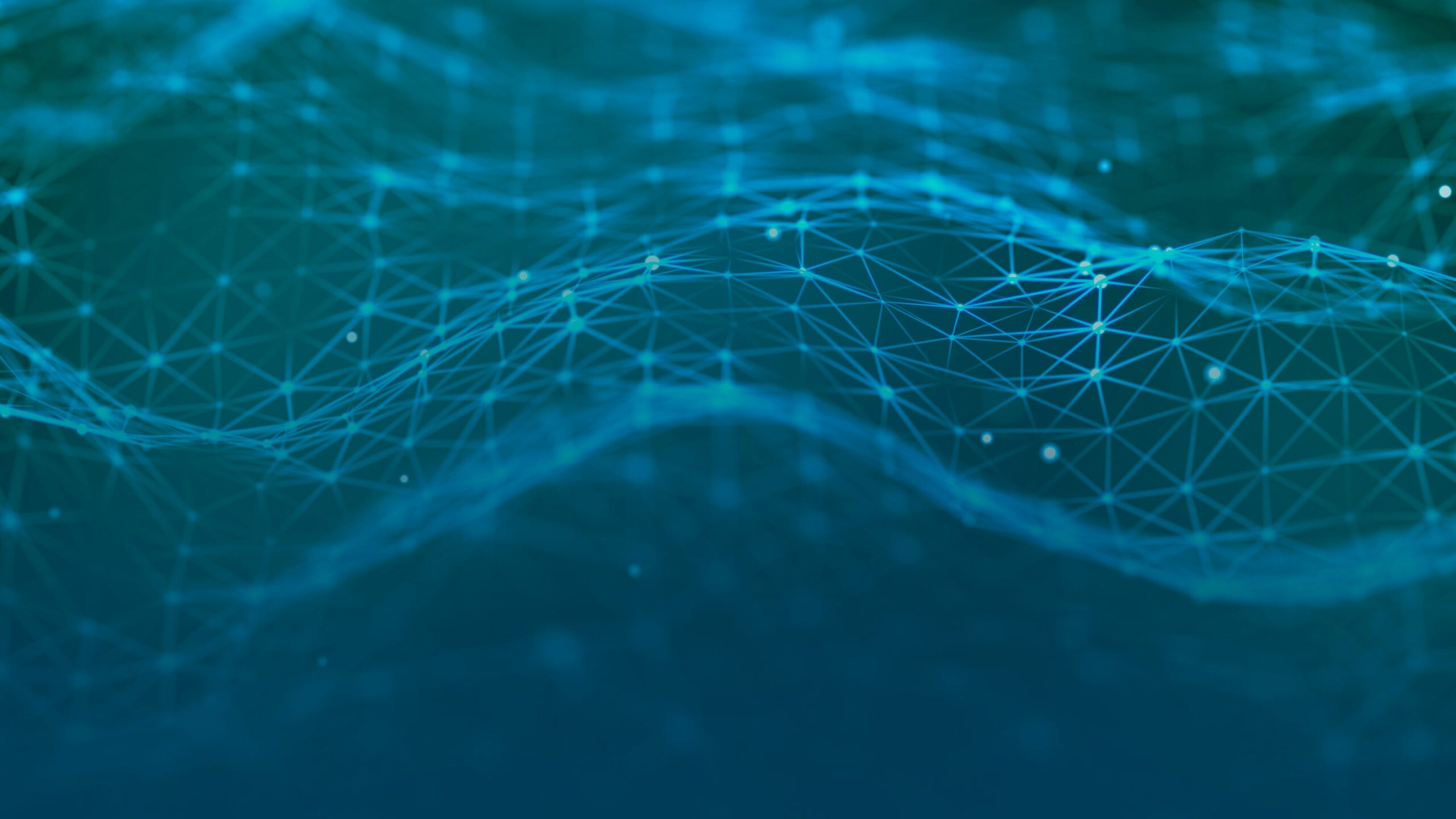
Infrastructure for Creating Digital Twin
Type of technology
Description of the technology
Basic elements
- IoT sensors: Devices for collecting real-time data from physical objects.
- Communication systems: Protocols and networks that enable data exchange between sensors and analytics platforms.
- Analytics platforms: Real-time data processing and analysis tools.
- Simulation software: Process and system modelling tools.
- Computing servers: High-performance units for processing large volumes of data.
Industry usage
- Manufacturing industry: Create digital replicas of production lines to optimise processes.
- Transportation: Modelling and optimisation of logistics and transportation routes.
- Construction: Monitor the condition of buildings and infrastructure in real time.
- Energy: Power grid simulation and monitoring.
- Resource management: Optimising resource use in agriculture and heavy industry.
Importance for the economy
The digital twin infrastructure enables companies to create exact replicas of their production processes to optimise operations, predict failures, and manage resources efficiently. It enables companies to minimise operating costs, improve efficiency, and innovate without the risk of disrupting actual operations.
Related technologies
Mechanism of action
- The infrastructure for creating a digital twin is based on the collection of data from real-world objects using IoT sensors and other measurement devices. The data is then sent to central analytical systems that process it in real time and create a dynamic virtual model of the object. The model is updated as conditions change, making it possible to map the actual state of the object, predict its behaviour, and optimise its processes.
Advantages
- Optimisation: It enables optimisation of production and operational processes.
- Risk minimisation: It enables testing of changes without disrupting actual operations.
- Predicting failure: Detecting and predicting potential failures.
- Cost savings: Reduction of operating costs through precise resource management.
- Faster innovation: Testing new solutions in a virtual environment.
Disadvantages
- High implementation costs: Significant financial investment in infrastructure.
- Complexity of integration: Problems with combining data from different sources.
- Data security: Risk of security breach of sensitive data.
- Lack of competence: Shortage of specialists capable of designing and maintaining infrastructure.
- Update problems: Maintaining the accuracy of models in a dynamically changing environment.
Implementation of the technology
Required resources
- IoT sensors: Devices to monitor the condition of physical objects.
- Network infrastructure: Communication networks for real-time data transmission.
- Simulation software: Tools for creating and updating models.
- Analytics platforms: Data processing and analysis tools.
- Teams of engineers: Specialists responsible for infrastructure design and maintenance.
Required competences
- Data engineering: Design and management of data processing infrastructure.
- Project management: Organising and running complex technology projects.
- Programming: Knowledge of data analysis languages, such as Python and Java.
- Cybersecurity: Protecting the data collected and processed by IoT sensors.
- Simulation modelling: Creation and maintenance of digital replica models.
Environmental aspects
- Energy consumption: High energy demand of servers and analytical systems.
- Emissions of pollutants: Indirect emissions from electricity consumption.
- Raw material consumption: High wear of electronic components.
- Recycling: Difficulties in recycling complex computing devices.
- Waste generated: Problems with disposal of used IoT equipment and sensors.
Legal conditions
- Data protection: Regulations for the processing of sensitive data, such as GDPR.
- Industry regulations: Standards for the use of the digital twin in the industrial sector.
- Intellectual property: Patents for simulation technology and modelling.
- Data security: Regulations for the protection of data from unauthorised access.
- Export regulations: Export control of digital twin technology.