Business aspects of Big Data processing include strategies, business models, and management methods that enable companies and organisations to derive business value from analysing large volumes of data. They include using data to improve decision-making processes, optimise operations, create new products, and tailor offers to individual customer preferences. This requires an understanding of both the technological opportunities and challenges of using Big Data.
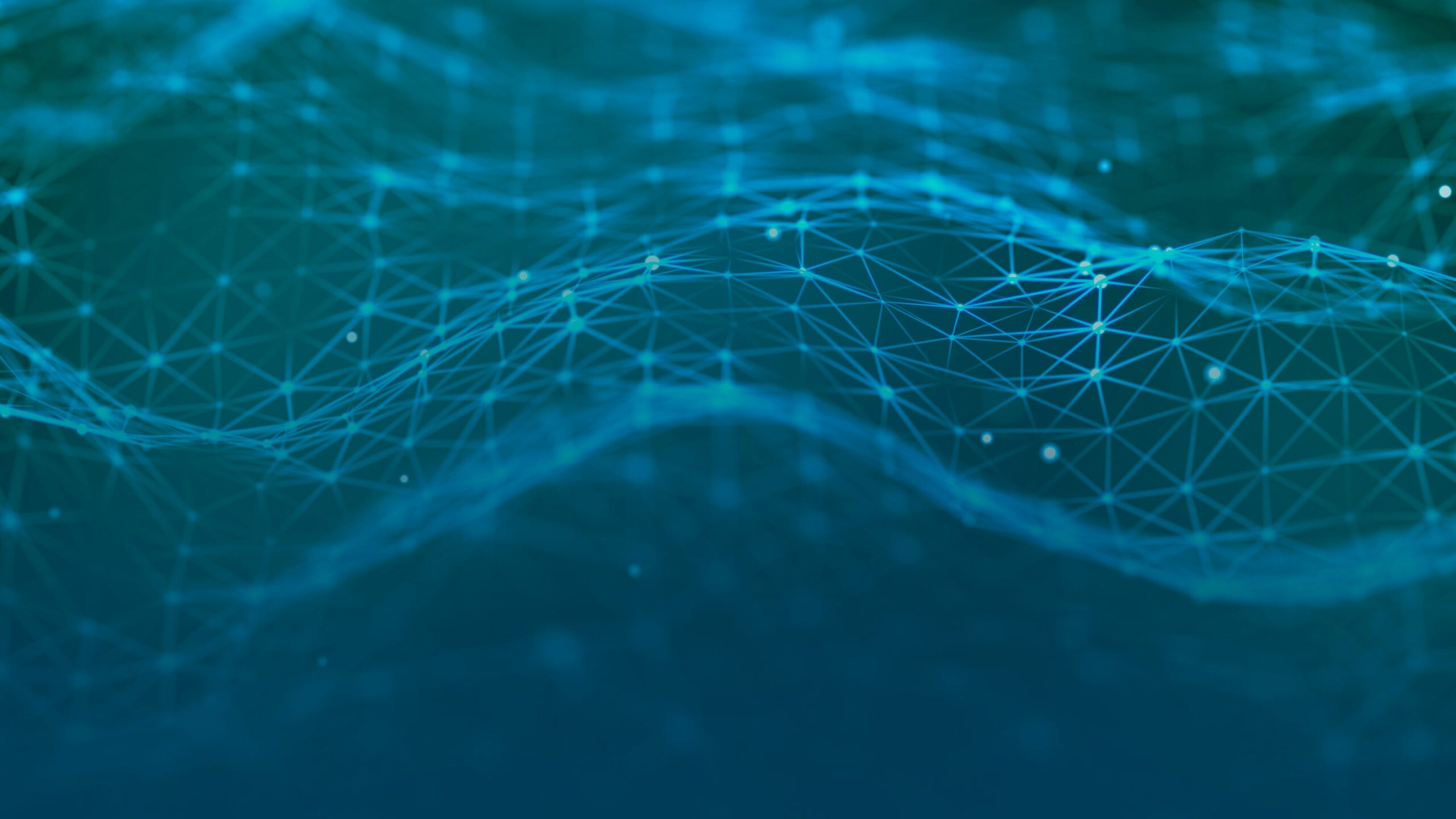
Business Aspects of Big Data Processing
Type of technology
Description of the technology
Basic elements
- Data analysis strategies: Action plans based on analysis of large volumes of data.
- Data-driven business models: Business strategies that include data as a key resource.
- Cost-benefit analysis: Evaluating the cost-effectiveness of Big Data solution implementations.
- Risk management: Identification and management of data risks.
- Personalisation: Customisation of products and services based on data analysis.
Industry usage
- Customer segmentation: Grouping customers based on their preferences and buying behaviour.
- Credit risk assessment: Predicting customers’ creditworthiness based on financial data.
- Competitive analysis: Identification of competitive advantages and market weaknesses.
- Inventory management: Optimising inventory levels based on demand.
- Product personalisation: Creating customised offers based on customer data analysis.
Importance for the economy
The business aspects of processing large data sets are crucial to the development of innovative business models and the optimisation of companies’ operational processes. Using data as a strategic resource enables the creation of new revenue streams, optimisation of costs, and better adaptation to market demands. Data analysis helps companies gain a competitive edge and make better strategic decisions.
Related technologies
Mechanism of action
- The business aspects of processing large data sets are based on using the results of data analysis to optimise business processes. It starts with defining business objectives. Then, the appropriate data processing methods are selected to obtain useful information. The results of the analysis can be used to develop business strategy, personalise services, forecast trends, and make strategic decisions.
Advantages
- Increasing revenue: Creation of new revenue streams by leveraging data.
- Operational optimisation: Better management of resources and processes.
- Customer personalisation: Personalising offers and products based on customer preferences.
- Faster decision-making: Reducing response time to market changes.
- Better risk management: Identification of potential risks based on data.
Disadvantages
- High implementation costs: Significant financial investment in analytical solutions.
- Risk of data breaches: Potential risks of data security and privacy.
- Lack of competence: Shortage of specialists capable of implementing data-driven strategies.
- Overreliance on data: Excessive dependence on the results of the analysis can lead to wrong decisions.
- Regulatory problems: Risk of non-compliance with data protection regulations.
Implementation of the technology
Required resources
- Analytics infrastructure: Servers and analytical tools for processing large data sets.
- Data sets: Data on customers, market, operations, etc.
- Analytics software: Tools such as Apache Hadoop and Tableau.
- Analysis teams: Specialists in data analysis and interpretation of results.
- Cybersecurity systems: Protecting data from unauthorised access.
Required competences
- Business analysis: Understanding the impact of data analysis results on business processes.
- Business modelling: Ability to design data-driven strategies.
- Data management: Processing and organisation of large data sets.
- Programming: Knowledge of languages used in data analysis, such as Python and R.
- Risk management: Identifying and assessing data risks.
Environmental aspects
- Energy consumption: High energy consumption of servers and analytical systems.
- Emissions of pollutants: Emissions from high electricity consumption.
- Raw material consumption: High demand for specialised electronic components.
- Waste generated: Problems with recycling complex computing devices.
- Recycling: Difficulties in recovering materials from advanced equipment.
Legal conditions
- Data protection: Regulations for the processing of customer data, such as GDPR.
- Industry regulations: Standards for the use of data in the financial and marketing sectors.
- Intellectual property: Patents for analytical algorithms and business models.
- Data processing regulations: Standards for protecting sensitive data.
- Occupational safety: Regulations for working with sensitive data.