Machine learning (ML) is a technology that enables computer systems to learn on their own from available data and gradually improve their performance without the need for manual programming. It is based on algorithms that analyse data, identify patterns, and use patterns to make decisions or predictions. Representational machine learning (RML) is an approach that automates the identification of relevant features in input data. The process is iterative and enables continuous optimisation of the models.
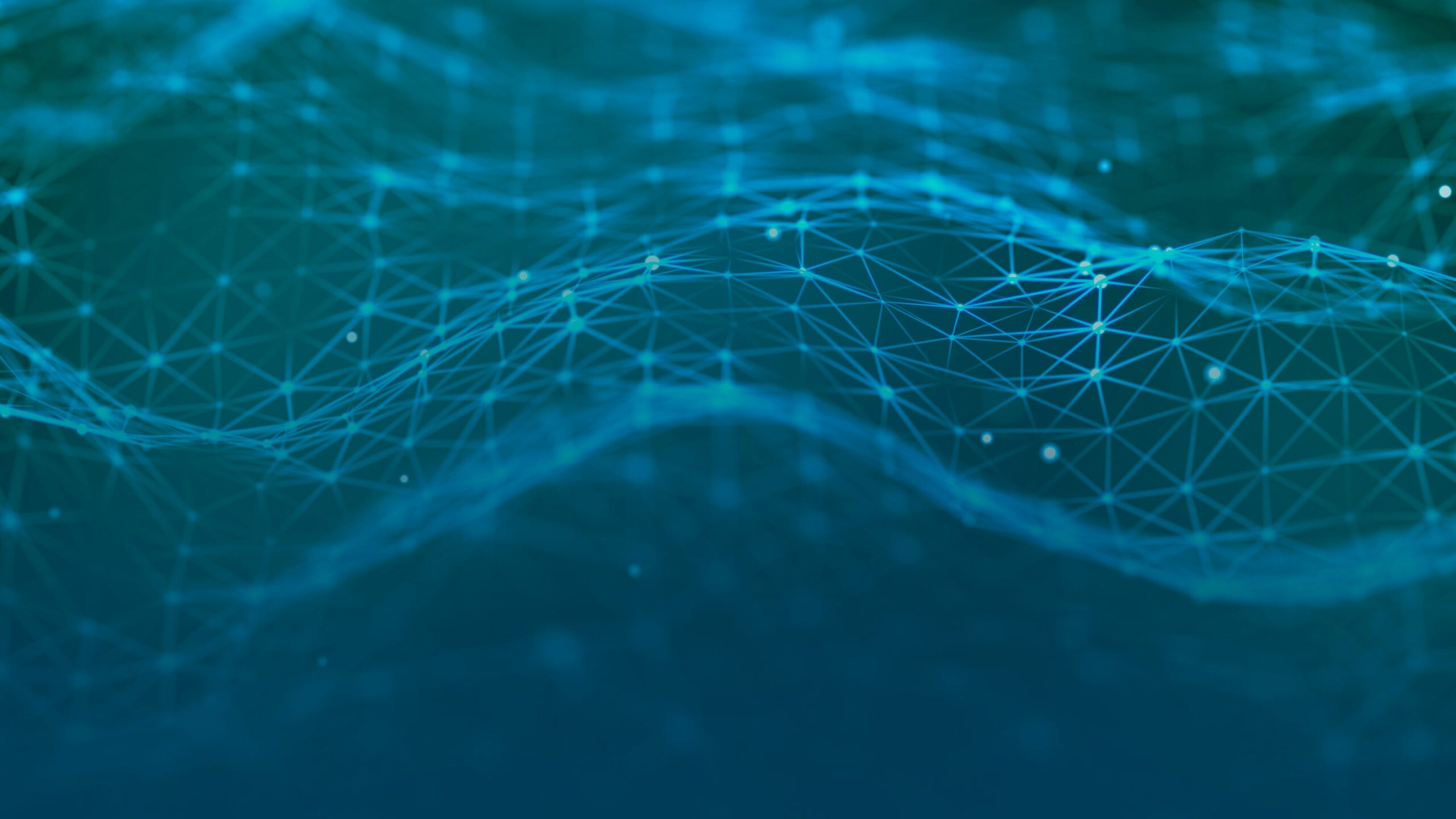
Machine Learning (ML/RML)
Type of technology
Description of the technology
Basic elements
- Learning algorithms, such as regression, SVM, and neural networks.
- Data sets: Data for model training, testing, and validating.
- Cost function: A measure of the error between predicted and actual results.
- Training process: The process of fine-tuning the model to achieve the best results.
Industry usage
- Medicine: Disease detection, medical image analysis, and therapy optimisation.
- Finance: Fraud detection, credit risk analysis, and investment automation.
- Trade: Personalising offers, forecasting sales, and analysing customer preferences.
- Industry: Optimisation of production processes and predictive maintenance of machines.
- Marketing: Segmenting customers, personalising advertising campaigns, and analysing purchase behaviour.
Importance for the economy
Machine learning is of great importance for automating processes in industry, services, and government. It improves operational efficiency, reduces costs, and enables the development of advanced products and services. It is a key component of the digital transformation that is boosting the competitiveness of companies and sectors.
Related technologies
Mechanism of action
- Machine learning involves analysing input data to detect patterns, which algorithms transform into a predictive model. Based on these patterns, the model makes decisions or predictions. The process of training ML models is iterative – the model is gradually improved by adjusting parameters to minimise the error in predictions.
Advantages
- Improved operational efficiency: Fast data processing and analysis automation.
- Precise forecasts: ML models can predict behaviour based on available data.
- Time and cost savings: Automating complex decision-making tasks reduces the need for manual work.
- Adaptation to new data: Algorithms learn and improve their performance based on new information.
Disadvantages
- Data errors: Inadequate input data can lead to erroneous results.
- Lack of transparency: Some algorithms, such as deep neural networks, are difficult to explain.
- Privacy issues: The use of large collections of personal data may violate users’ privacy.
Implementation of the technology
Required resources
- Data: High-quality data sets to train models.
- Computing power: Servers or cloud access for ML algorithm processing.
- Specialised software: Tools for building and training models, such as TensorFlow and PyTorch.
- Team of specialists: Data experts and AI engineers.
- IT infrastructure: Stable systems for processing and storing large data sets.
Required competences
- Knowledge of ML algorithms: Ability to select and optimise algorithms for specific applications.
- Data analysis: Ability to process, clean, and analyse input data.
- Programming: Knowledge of programming languages, such as Python and R.
- Cloud management: It is used to support large models in cloud environments.
- Understanding business problems: Ability to translate data into tangible business results.
Environmental aspects
- Energy consumption: Training ML models requires a lot of energy resources.
- Emissions of pollutants: The computing infrastructure used in ML can generate CO2 emissions.
- Waste generated: Upgrading IT infrastructure to ML can generate electronic waste.
- Recycling: Effective methods of recycling for used IT infrastructure are needed.
- Raw material consumption: The production of servers and ML equipment requires significant amounts of raw materials.
Legal conditions
- Legislation governing the implementation of solutions (e.g. AI Act).
- Environmental standards: Regulations for reducing emissions from data centres.
- Data security: Data protection regulations (e.g. GDPR).
- Intellectual property: Rules for the protection of algorithms and data.
- Export regulations: Regulations governing the export of ML technology.